Data flows across the supply chain from an increasingly diverse range of sources. The internet of things has allowed companies to augment their planning and inventory management systems with real-time information on product location obtained from RFID tags and vehicle telematics systems. They know more about customer behavior by tracking their purchasing and browsing activities on-line. They have new information on the performance of their own products, which can report their status over the internet.
These advances have already brought a host of benefits, allowing products to be tracked more accurately, delivered more quickly and stored more efficiently. Now the supply chains of many businesses are set to undergo an even more radical transformation, with the advent of technologies that can sift through all this data to identify hidden opportunities to improve supply chain performance and then make the smart decisions required to capture those opportunities.
Today, it is consumer-facing companies that are at the forefront of this revolution. British grocery retailer Ocado, for example, is using artificial intelligence and machine learning techniques to ramp up the performance of its logistics operations. As technology director Paul Clarke told a recent conference: “If you want to build really smart systems, you are going to have to feed them with a huge amount of data.” As he put it: “Artificial intelligence literally eats data science for breakfast.”
Ocado collects data from across its business, with clickstream data from mobile apps and its grocery website, business data, and what Clarke calls “the data exhaust” from the automated warehouse and the data streamed back in real time from vans as they make deliveries across the country. This is complemented by unstructured data from customers, such as emails, telephone calls and social media posts.
Sense, forecast, react
“We use this data to drive our forecasting, to drive predictive analytics, to optimize our platform in real time and to monitor its performance. But we also use it to feed our voracious smart systems,” Clarke told the conference. For instance, Ocado uses data to improve the layout of its warehouses by using machine learning algorithms. The data tells the digital team how long it takes to pick certain items and how the orders flow through the warehouse, allowing them to plot the most effective routes to picking goods. If there is a fragile product it can be moved to a different location to make it easier to pick.
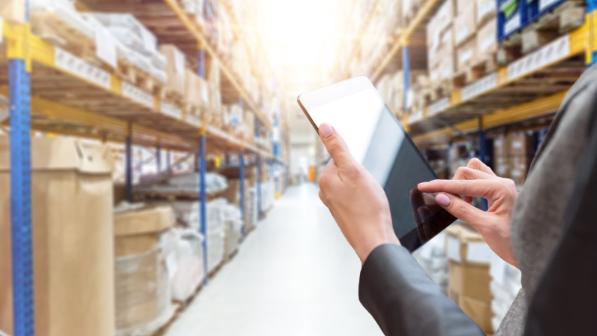
The retailer stores data about 5,000 business transactions that occur in its fulfilment centers every second, using the Cassandra NoSQL database. This type of modern database makes it possible to store many different types of data, both structured and unstructured. It is an important enabling technology for the new generation of intelligent supply chains. The older relational databases that businesses have been using since the 1980s struggle to handle today’s complex and diverse data streams.
Not just for the unicorns
It isn’t just the world of fast-moving consumer goods that stands to benefit from data-driven decision-making. Consultancy Accenture cites the example of an industrial equipment maker that used advanced analytics techniques to find failure patterns hidden in its warrantee claims, thereby improving the reliability of its products.
Carmaker BMW is already working on a “connected logistics” concept for its upstream supply chain, using automation, analytics and connectivity to build a supply chain that is faster, more robust and more able to respond quickly to problems or changes in demand. “In the long term, we want to move away from central steering towards the self-steering of objects in the supply chain,” BMW Group’s board member for production, Oliver Zipse, told a German automotive conference last year.
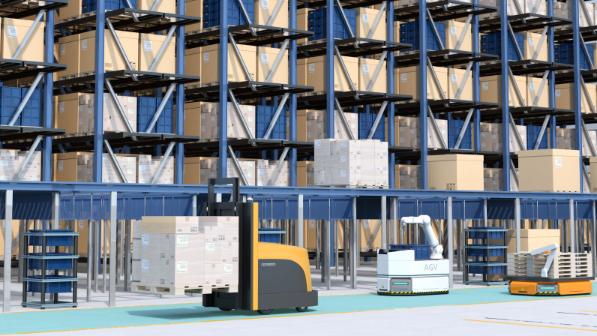
Today, these examples are the exception rather than the norm, however. Accenture found that only 17 percent of companies report having already implemented analytics in one or more parts of their supply chain. But it also found that many companies are on the verge of making significant investments in big data analytics.
First, get the right data
The first barrier to the widespread use of big data is the difficulty companies have in actually finding the right information about their stock and supply chains. There are examples of companies that know they have hundreds of thousands of stock keeping units, but have no dimensional data about them – they don’t know whether each product is tiny, small, medium, large or enormous.
A company without in-depth data about its own operations will struggle to get the most out of big data. There are fears that companies may jump into big data because they have been told it is the future. But first, they need to be clear about exactly what are they trying to achieve. How will big data analysis make their business better and will it help them serve customers better?
Accenture believes these companies can learn lessons from the small group of businesses that have generated a high return from their investments in big data analytics. The high performers tend to use data analytics across the whole enterprise and embed them into supply chain operations to improve decision-making. They use data systematically and strategically across their whole organization rather than on an ad hoc basis for specific situations. They also hire staff with a combination of analytical skills and business acumen who can produce actionable insights from data.
Why go looking for the latest logistics stories and insights when you can have them delivered right to you?
Handling uncertainty
There are other significant complications on the path to using big data to make supply chains more intelligent. Çağrı Haksöz, a researcher on supply chain, risk and operations management at Sabanci School of Management at Sabancı University in Turkey, says big data’s ability to predict events depends on the underlying stability of the supply chain. “First you have to understand the context, the environment and the setting in which you are taking a decision. It may be a stable environment – Northern Europe is different from Southern Europe, while the Balkans are different again,” he says.
Handing supply chain decision-making over to a mathematical model, however sophisticated, risks creating overconfidence in managers, notes Haksöz, which can lead to real problems if the model fails to predict an uncertain event. One way to improve the way models handle the unexpected, he suggests, is to collect as much information as possible on “near misses” or “close calls” that are avoided, but which might lead to a disruptive event – such as poor driving that nearly leads to a truck crashing.
“In the supply chain, if you are managing a fleet, the information you might collect on those near misses could be the drivers behaving differently on different routes, or reckless drivers who take reckless risks,” he says. If the behavior of their driving patterns could be understood through the internet of things or telematics without interfering with the driver’s behavior, this could reveal any problems that potentially become a major disruption.
The same approach can be applied to more prosaic events, he notes, like analyzing the behavior of customers to determine why they come close to buying a product but pull out at the last minute. “If the behavioral pattern of the customer could be analyzed, you could do something to ensure there is a successful purchase,” he says.
Industrial supply chains can offer similar telltale warnings, if the right data collection and analysis approaches are there to spot them. Vibration or temperature monitoring sensors attached to machinery can provide early warning that a bearing is nearing the end of its life, allowing a replacement to be ordered, for example.
External data sources can help as well. Consumer goods companies can learn about upcoming demand by monitoring social media data generated by the customers. In the industrial sector, a commodity price spike might be an early warning indicator of an upswing in demand for new equipment or spare parts, as producers ramp up production in response.
Overall, says Haksöz, big data is suitable for predicting “white swan” events, which are everyday occurrences, and monitoring near misses can help predict “gray swan” events, which are uncommon but foreseeable. Big data will always struggle to handle truly unpredictable “black swan” events, however, which are not foreseeable but can occur in extreme circumstances.
Algorithmic decision-making can have unexpected consequences even when the world performs as expected. In the financial markets, for example, “flash crashes” have occurred when thousands of automated trading progams react instantly, and in the same way, to market events, causing wild swings in asset prices. In logistics, similar effects have been seen when automated navigation tools send many vehicles down the same narrow streets to avoid congestion, causing their own “algorithmic traffic jam.”
Adopting big data analytics requires significant investment in technology, data collection and skilled staff to make sense of the data. It also requires a transformation in the way senior managers understand the future of their businesses. Intelligent supply chains need an abundant supply of human intelligence and a significant commitment from company leaders. — David Benady
Published: February 2017
Images: iStock