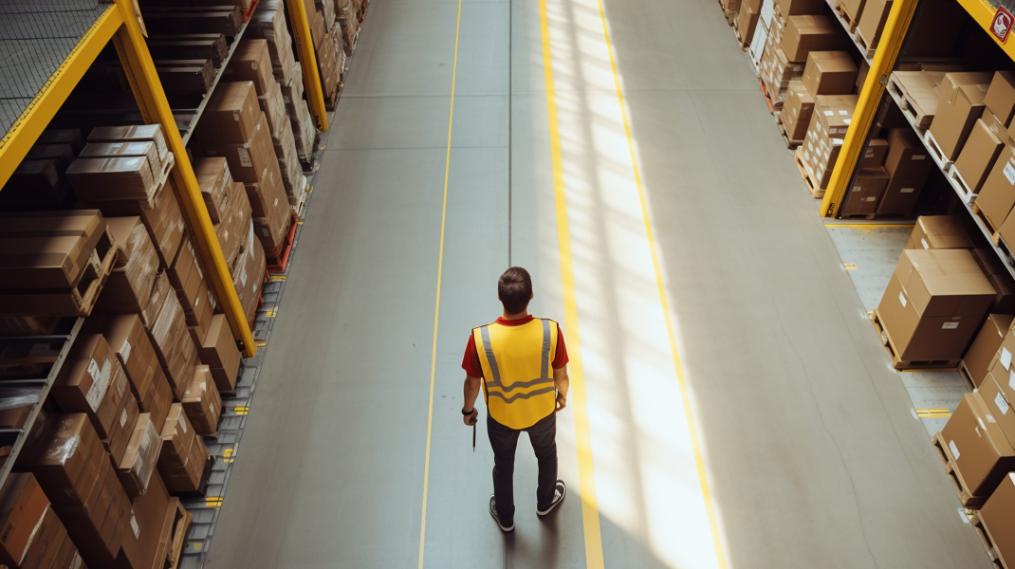
Logistics Use Cases: People - Operations
People and Operations Applications
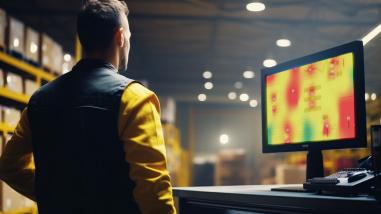
Heatmaps
Where are the bottlenecks and activity hotspots in the warehouse? Computer vision-based heatmap analysis can help evaluate workflow patterns inside a facility and outside in the yard with contactless, non-invasive methods.
A computer vision-based heatmap uses surveillance camera video feeds to analyze the frequency and duration of movement in different areas by both people and vehicles. Typically, a color-coded overlay is used on original images with, say, red indicating heavy activity in one area and white indicating no activity in another. This helps managers identify bottlenecks and other inefficiencies such as overutilized and underutilized areas. This information can be used to place inventory and equipment in the best locations and increase the efficiency of operations. To measure the effectiveness of any changes, heatmaps can be compared over time.
Headquartered in Switzerland, Viso offers movement heatmap crowd detection to identify human movement trajectories in public spaces and in logistics operations. This computer vision solution can improve operational efficiency by tracking changes over time, finding anomalies and detecting hot spots and bottlenecks.
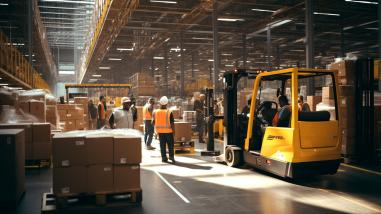
Head Counting
Warehouse managers need to have enough people to do the required tasks at any given time. If there are too few, tasks may not be completed on time. If there are too many, the company incurs unnecessary staffing costs. And it’s essential to comply with safe occupancy levels.
Counting people in a defined space, however, can be a tedious, repetitive task that takes up a lot of time. Instead, computer vision systems can be used to count people and vehicles in any logistics facility 24/7. This enables logistics organizations to keep track, and analysis of this visual data, helps management decide the optimal number of people to perform specific tasks and comply with occupancy requirements.
Indian startup AIVID has created an AI software using surveillance cameras for automated detection and counting, even distinguishing age and gender, to provide operational insights and patterns for businesses.
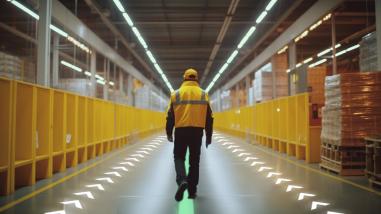
Pick Path Optimization
Products in a warehouse must be picked quickly, accurately, and efficiently so people who do this task (pickers) must take the best and most optimal routes during their shift. Their activity can account for a substantial percentage of warehouse operations tasks, so optimizing the pick path provides quantifiable savings in operating costs. It ultimately also results in higher customer satisfaction.
Algorithms alone cannot guarantee success in every picking scenario, but companies are more likely to achieve pick path optimization when computer vision is used.
Camera video feeds inform machine-learning algorithms to identify patterns and trends in the data, making recommendations to change workflows; for example, guiding people to walk shorter distances but still complete their picking tasks by moving the location of some equipment or supplies. These feeds can also show the benefit of changing the sequence in which certain tasks are completed. If a heatmap has revealed high activity in a particular area of the warehouse, this solution may help to understand the workflow in that area so that changes can be made to resolve the bottleneck.
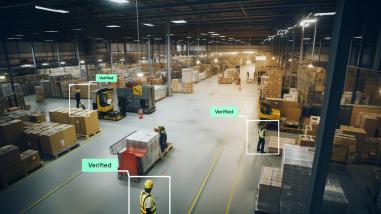
Access Control
Security surveillance using a traditional camera setup requires human interaction and effort to scan through many recordings. Compare this with an intelligent surveillance system which not only records video footage but also leverages advanced algorithms and techniques for real-time motoring, analysis, and detection. Backend AI systems help to rapidly process the video footage and provide rich information which can be used to increase safety and reduce theft.
A computer vision-enabled camera system can act both as a view-only platform and to detect unauthorized entry or intrusion into restricted areas. By analyzing the video feed, the system can identify when someone crosses a virtual boundary or enters a forbidden space. Alerts can be generated immediately, allowing security personnel to respond promptly. Additionally, the system’s algorithm can recognize patterns of normal activity and behavior within the monitored environment. When an abnormality is detected, such as a person loitering or running in a restricted area, the system can generate alerts, enabling proactive intervention.
Challenges of Implementation
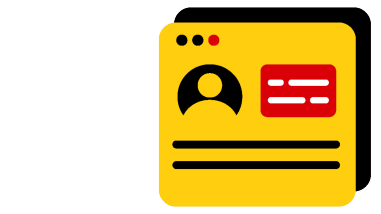
Challenge 1: For privacy reasons, companies are usually disallowed from monitoring workforce activity solely for that purpose. Data from computer vision monitoring should be used to optimize operations, and this should be explained to the workforce.
Challenge 2: If workplace surveillance methods to track workforce activity are perceived as invasive, it can reduce morale, increase work-related stress, and cause counterproductive work behaviors.